Artificial intelligence has given birth to two prominent paradigms that have emerged as the giants of innovation in the field of technology- Large Language Models (LLMs) and Generative AI.
Although falling under the umbrella of artificial intelligence, both of them serve purposes that are quite distinct, are built on different architectures, and have unique applications.
However, to extract and utilize the full potential of these technologies, it is crucial to understand the key differences between them. And that is what we will delve into in this comprehensive blog.
Let’s dive into the world of LLMs and Generative AI, dissecting their architectures, exploring real-world applications, and pondering their implications for the future of artificial intelligence.
Explaining LLMs
An LLM represents the peak of Natural Language Understanding (NLU) and generation within artificial intelligence. As a class of deep learning models, LLM is designed to process and generate human language text with unparalleled proficiency.
What Is Generative AI?
Generative AI is an intricate subset of artificial intelligence that represents the pinnacle of creative computation. Generative AI seeks to flawlessly imitate human-like creativity and imagination by generating original and meaningful content.
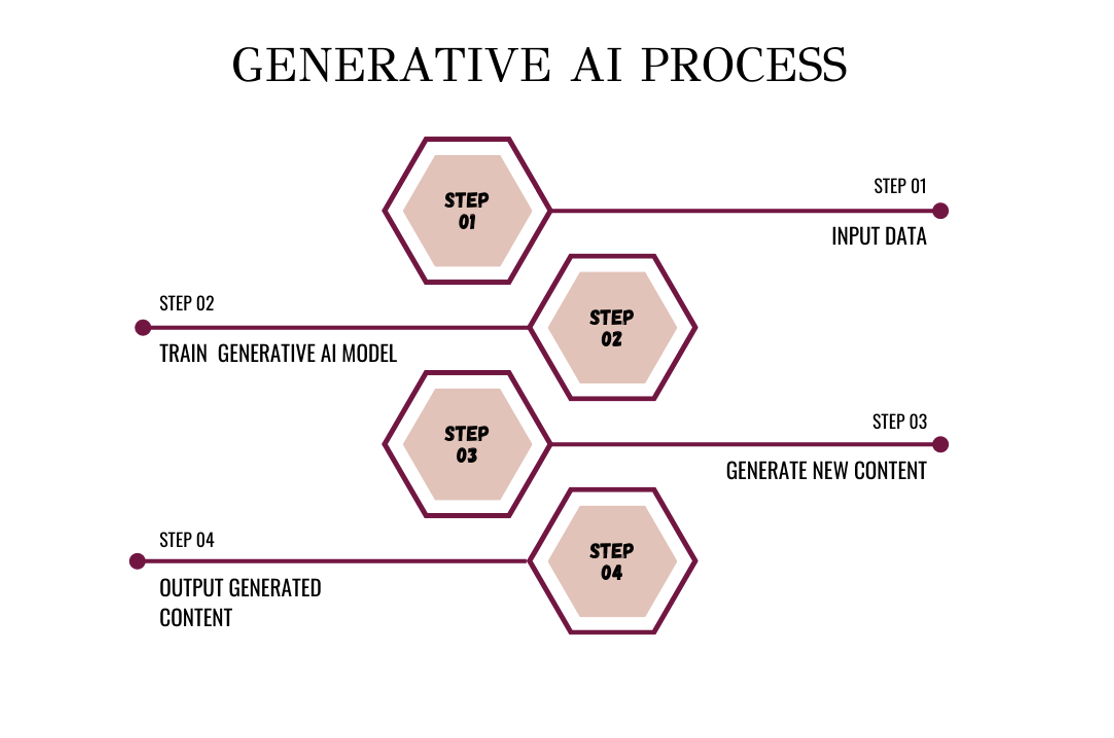
The Key Features Differentiating LLM and Generative AI
Both LLMs and Generative AI are powerful technological advancements, but as we discussed before, they are designed for varying purposes and possess unique traits that set them apart.
What are these traits? Let’s look at those now.
LLMs
Language-Centric: LLMs are principally focused on understanding and processing human language. They excel in tasks related to NLU and generation.
Pre-training on Text Corpora: LLMs undergo extensive pre-training on huge amounts of text corpora. This pre-training phase arms them with language comprehension capabilities, qualifying them to understand and generate text.
Transformer Architecture: LLMs are built on the Transformer architecture, that facilitates effective parallel processing of sequential data, making them extremely competent for language-related tasks.
Applications: LLMs find applications in NLU responsibilities like sentiment analysis, named entity recognition, query answering, and language translation. They are used in chatbots, virtual assistants, and content generation, too.
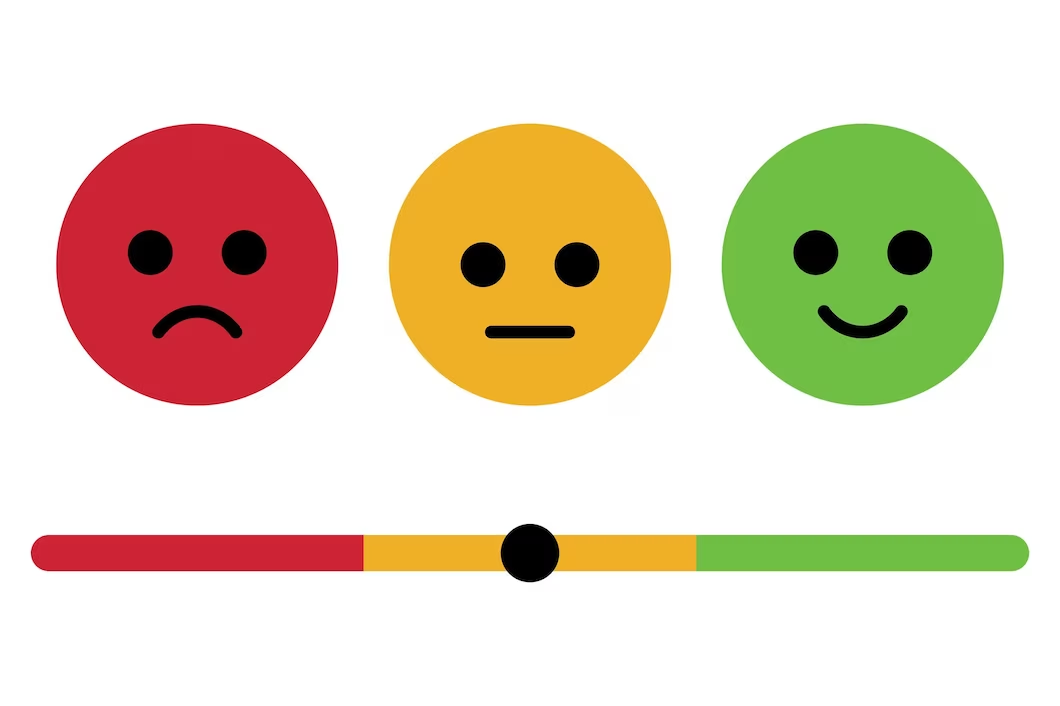
Multilingual: LLMs can comprehend and generate text in multiple languages, which helps break down language barriers and enables cross-lingual applications.
Transfer Learning: LLMs can be pre-trained on a general language comprehension task and fine-tuned for definite downstream tasks. The transfer learning approach decreases the need for extensive labeled data.
State-of-the-Art Performance: LLMs consistently perform well on numerous language-related benchmarks and challenges, showcasing their efficiency.
Generative AI
Creativity-Centric: Generative AI revolves around creativity and innovation. It is intended to create new data, including new designs and new content, rather than concentrating merely on language processing.
Variety of Models: Generative AI incorporates various generative models, including Variational Autoencoders (VAEs), autoregressive models, and Generative Adversarial Networks (GANs). These models enable creativity across several domains.
Probabilistic Modeling: Generative AI has many generative models that operate based on probabilistic principles, which ascertain that generated content adheres to certain statistical distributions.
Diverse Outputs: Generative AI can produce a wide range of outputs, including accurate images, tune compositions, textual content, and much more. It is not just restricted to language-related tasks.
Applications: Now, we know that Generative AI has applications in art generation, melody composition, content creation, and image generation. Additionally, it has applications even in scientific discovery by suggesting new molecular structures.
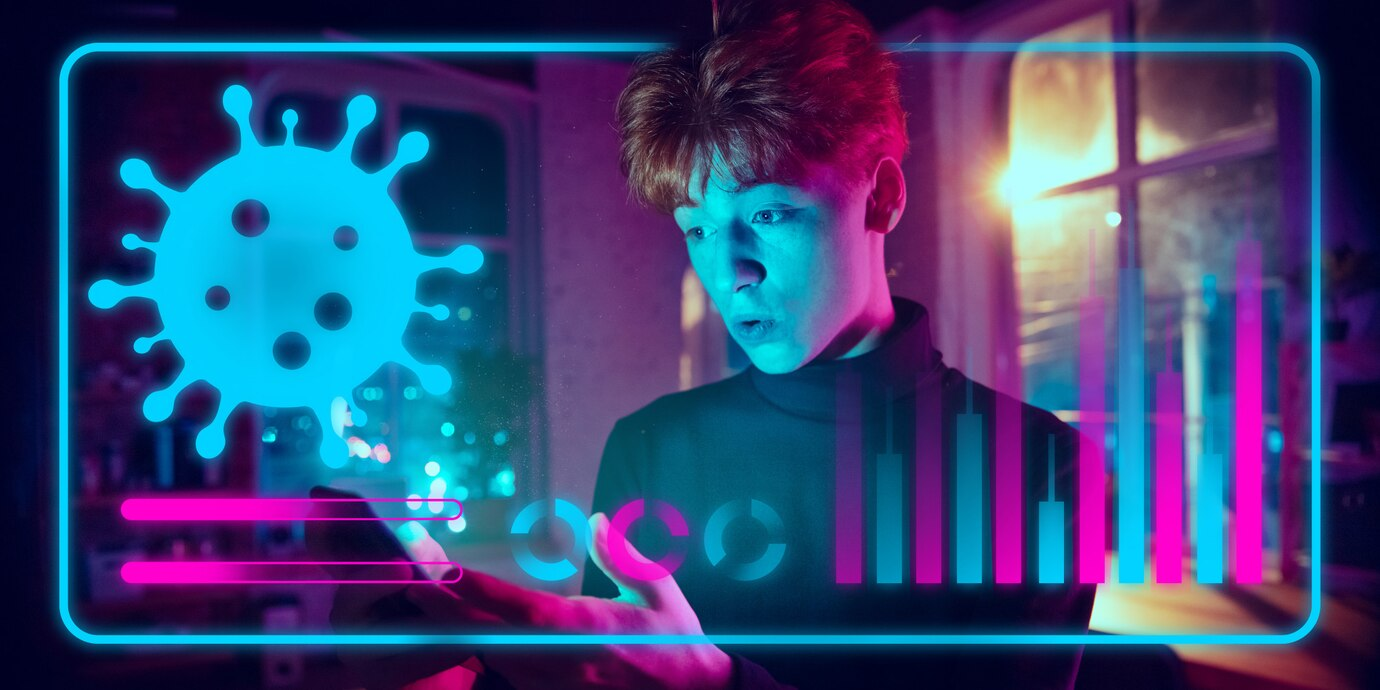
Automation: Generative AI can automate creative tasks, thus significantly reducing the time and effort required for tasks like art creation, content production, and music composition.
Ethical Concerns: There have been some ethical concerns, especially as it has been used for misinformation, deepfake creation, and malicious purposes. It is clear that ethical considerations are crucial in its application.
In summary, while both LLMs and Generative AI are artificial intelligence technologies, they cater to different domains and objectives. LLMs are language-centric and excel in language understanding and generation, while Generative AI focuses on creativity and innovation, producing diverse forms of content beyond text. Understanding these distinctions is essential for choosing the right technology for specific applications.
Some Real-World Examples of the Two Concepts Under Artificial Intelligence
Exploring some real-world examples of both LLMs and Generative AI to better understand their practical applications:
Real-World Examples of LLMs
Chatbots and Virtual Assistants: Chatbots, the likes of those powered by GPT-3, and virtual assistants like Amazon’s Alexa leverage LLMs to engage in human-like conversations, answer queries, and help users with tasks.
Sentiment Analysis: LLMs are also used for sentiment analysis in tools utilized for social media monitoring. They analyze and grasp the sentiment behind user-generated content, assisting businesses in gauging customer views and emotions.
Language Translation: Services like Google Translate employ LLMs to provide accurate and context-appropriate translations across numerous languages. These models have significantly enhanced the quality of automated translations.
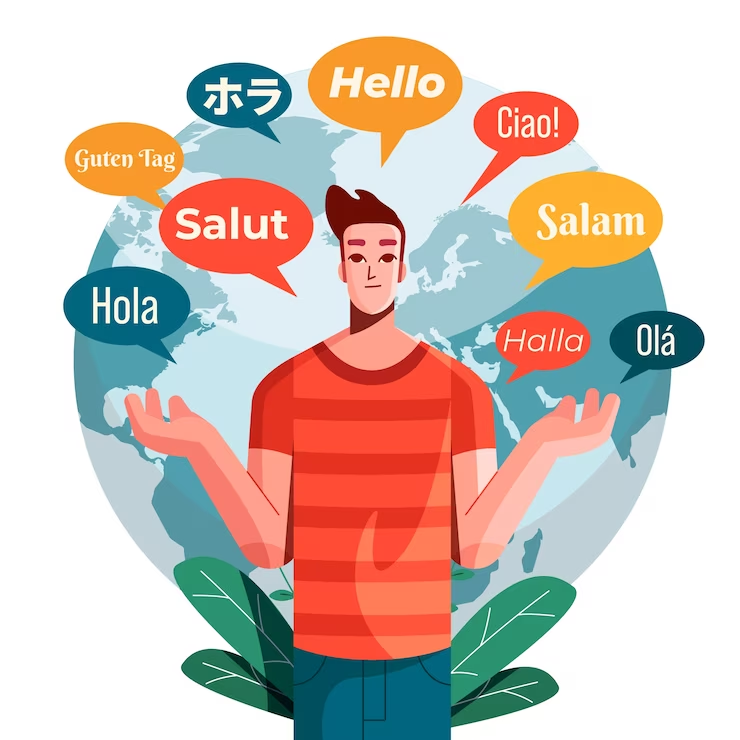
Content Generation: LLMs are also deployed in content generation tools, where they automate the writing of marketing copy, product descriptions, and news articles, hence saving time, resources, and effort for businesses.
Search Engines: Search engines like Google use LLMs to improve search results and recognize user queries better. They scan the language in web content to come up with relevant search results.
Question Answering Systems: LLMs are integrated into question-answering systems, allowing users to obtain accurate answers from large volumes of unstructured textual data, as seen in IBM Watson’s usage in healthcare.
Email Automation: Email automation tools use LLMs to draft personalized email responses, making email communication more effective.
Real-World Examples of Generative AI
Art Generation: GANs have been used to create art. For instance, “Edmond de Belamy,” an AI-generated portrait, was sold at an auction for $432,500.
Music Composition: Generative AI models like OpenAI’s MuseNet can compose melodies and music in various styles and genres, aiding musicians and composers in creating new pieces.
Deepfake Technology: Generative AI has been notoriously used in the creation of deepfake videos, where appearances and voices are manipulated to generate realistic but fake content, raising apprehensions about misinformation.
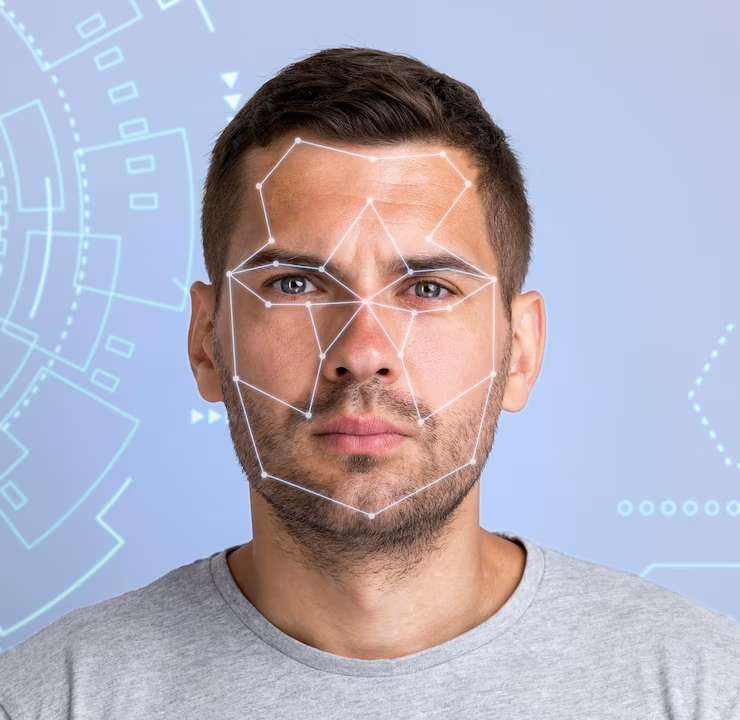
Data Augmentation: In machine learning, generative models are also used for data augmentation, generating additional training data to enhance model performance.
Game Development: Game developers leverage generative AI to create game assets, including themes, maps, characters, and levels. This allows them to speed up the game development process.
Drug Discovery: Generative AI is utterly helpful in drug discovery. It suggests potential molecular structures for new drugs and expedites research in the pharmaceutical segment.
Image Generation: GANs are used to create realistic images, from lifelike faces to artistic creations. NVIDIA’s GauGAN, for example, turns rough sketches into photorealistic scenes.
Final Thoughts
In conclusion, artificial intelligence is not only captivating but also highly diverse, offering a selection of specialized models and concepts to suit various needs. LLMs and Generative AI stand as two giants, each with its unique traits and applications.
Understanding the distinctions between LLMs and Generative AI is critical to making informed decisions when choosing the right technology for a given project.
As the segment of artificial intelligence continues to develop, we can anticipate even more advancements, pushing the limits of what LLMs and Generative AI can achieve.
Meanwhile, the key is to stay informed, harness the potential sensibly, and steer the intricate ethical considerations that arise with these transformative technologies. The journey of artificial intelligence discovery has merely just begun.