In the field of artificial intelligence, IT engineers and developers are focusing on machine learning. The AI branch requires data labeling to provide proper training to specific machines. But machine learning is still considerably new. As a result, companies engaged in the service face various challenges, such as the dataset’s quality, data privacy, workforce management, innovative tooling, and financial constraints. Finding the most effective data labelling solutions can help companies overcome the challenges that hamper them.
What is data labeling?
Data labeling is the process of detecting and tagging data with specific labels in the form of text, audio, videos, and images. Companies engaged in data labeling services use humans and computers to annotate data. A machine learning engineer predetermines the labels’ types and feeds the information into the machine learning models. It is critical to provide accurate labels to the datasets so that the machines can learn and identify individual pieces of data precisely and accurately. Machines are still computer-driven; thus, they require precise data input to have exact data output.
Data labeling process
Data labeling is one of the core parts of machine learning’s preprocessing workflows. The process provides structure to various types of data to make them more meaningful to the program. In addition, data labeling helps improve machine learning. Thus, it needs quality datasets and volumes of similar data to ensure that the machine can recognize objects appropriately. These inputs will be critical to the decisions that the devices will make later.
For example, in an image of a street scene, bounding boxes or image labels are applied to the various objects shown in the image. Cars can have bounding boxes in yellow. To differentiate the vehicles, trucks can have green boxes and orange boxes for buses. Traffic lights can be assigned red bounding boxes, while pedestrians can have blue boxes. The objects will also be given their generic names — car, truck, bus, traffic light, person, etc. The process can be repeated many times, depending on the requirements of the project and business use case.
Ensuring success in providing data labeling services
Your success will depend on your best practices and in handling each project accordingly.
- Ensure the collection of diverse datasets. There should be no bias when training a machine. You should provide all the possible data the project requires. For example, the project requires training a machine model for an autonomous car. Since a vehicle is not only used in the city, you should have datasets for all locations and situations where the vehicle will be used.
- Gather specific data. It is vital to provide data to train a machine for a particular purpose. For example, suppose your project is for a robot waiter. Make sure that you collect data from different restaurants where the robot waiter will be deployed.
- Provide specific guidelines. Although your annotators are all trained, it is still vital to have specific guidelines for each project.
Remember that data precision and accuracy are vital to data labeling. Therefore, understanding every project for data labeling and machine learning will ensure success.
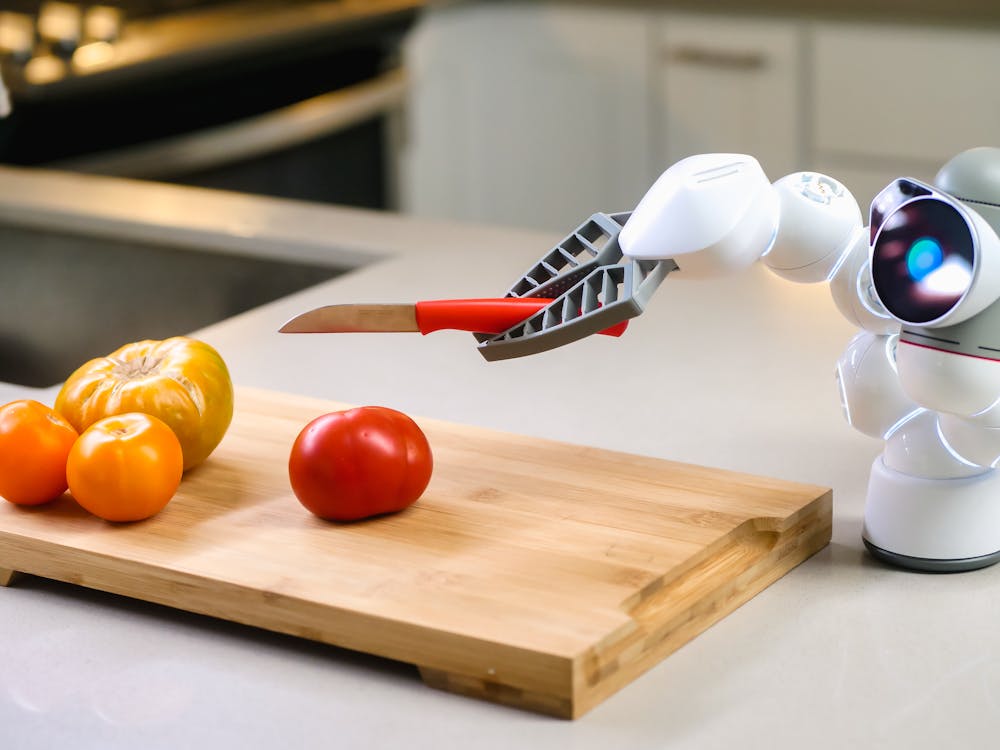
Image: https://www.pexels.com/photo/food-wood-man-people-9028912/